8.2. TensorBoard#
TensorBoard provides the visualisation and tooling needed for machine learning experimentation:
Tracking and visualising metrics such as loss and accuracy
Visualising the model graph (ops and layers)
Viewing histograms of weights, biases, or other tensors as they change over time
Projecting embeddings to a lower dimensional space
Displaying images, text, and audio data.
Logging#
In the csf_main.py we have used TensorBoard to:
log accuracy and loss values
show batch images
The SummaryWriter
class is your main entry to log data for consumption and visualisation by
TensorBoard. So, we import it:
from torch.utils.tensorboard import SummaryWriter
At the start, we initialise two instances of SummaryWriter
for train and testing, each logging in
their corresponding directories:
args.tb_writers = dict()
for mode in ['train', 'test']:
args.tb_writers[mode] = SummaryWriter(os.path.join(args.out_dir, mode))
We add new accuracy/loss by calling the add_scalar
function and add new images by calling the
add_image
function.
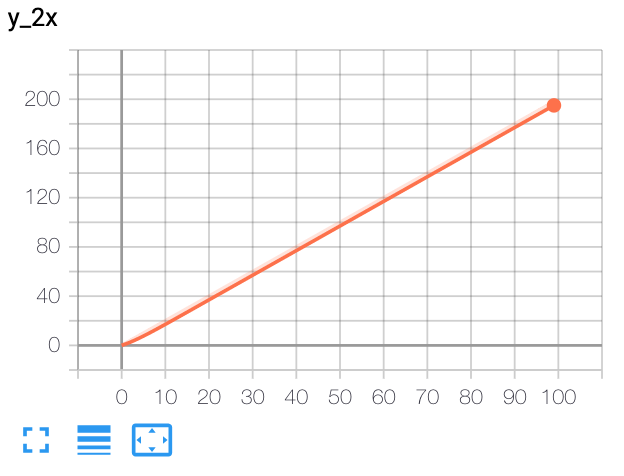
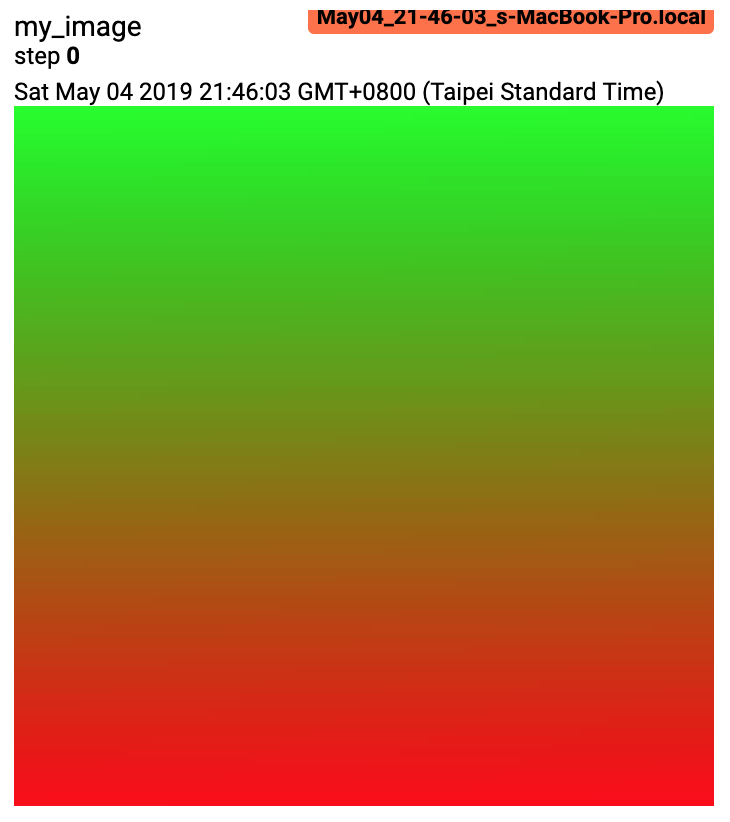
SummaryWriter
contains several add_<SOMETHING>
functions
(https://pytorch.org/docs/stable/tensorboard.html), most of them with a similar set of arguments:
tag (data identifier)
value (e.g., a floating number in case of scalar and a tensor in case of image)
step (allowing to browse the same tag at different time steps)
At the end of the programme, it’s recommended to close the SummaryWriter
by calling the close()
function.
Monitoring#
We can open the TensorBoard in our browser by calling
tensorboard --logdir <LOG_DIR> --port <PORT_NUMBER>
In our project, by default, the TensorBoard files are saved at csf_out/train/ and csf_out/test/
folder. If we specify the <LOG_DIR>
as the parent directory (csf_out/), TensorBoards in all
subdirectories will be also visualised:
This is a very useful tool to compare different conditions (e.g., train/test, different experiments) at the same time.
If there are too many nested TensorBoards, it might become too slow.
The value for <PORT_NUMBER>
is a four-digit number, e.g., 6006.:
If the port number is already occupied by another process, use another number.
You can have several TensorBoards open at different ports.
Finally, we can see the TensorBoard in our browser under this URL
http://localhost:<PORT_NUMBER>/